DeepSeek-R1: Revolutionizing AI Reasoning with Reinforcement Learning
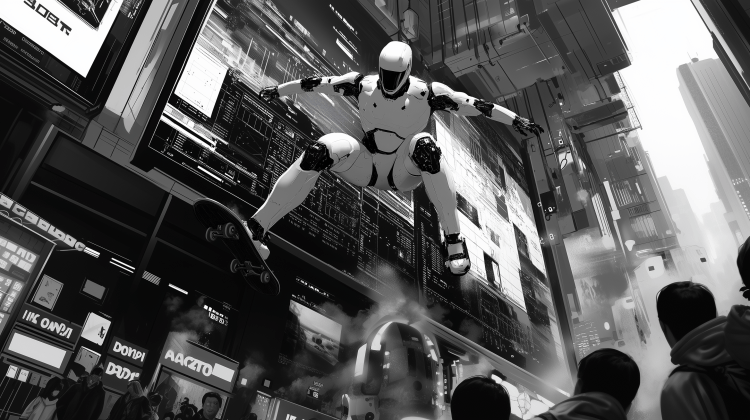
Introduction
The field of artificial intelligence has witnessed a significant leap forward with the recent release of DeepSeek-R1, a novel language model designed to enhance reasoning capabilities through reinforcement learning (RL). Traditionally, large language models (LLMs) have relied heavily on supervised fine-tuning (SFT) to improve their problem-solving abilities. However, DeepSeek-R1 challenges this approach by demonstrating that RL alone can cultivate strong reasoning skills, particularly in STEM-related fields. This paradigm shift has ignited competition among major AI players, including OpenAI, Meta, and Google, while raising fundamental questions about the future of LLM training methodologies.
The Problem DeepSeek-R1 Aims to Solve
Despite their advancements, current LLMs often struggle with complex reasoning, especially in mathematical and scientific domains. Standard models, trained primarily through SFT, exhibit limitations such as:
- Overfitting to training data, leading to a lack of generalization.
- Inability to generate novel reasoning strategies, as they rely on human-annotated datasets.
- Bias towards verbosity, producing unnecessarily long responses without improving accuracy.
DeepSeek-R1 addresses these issues by leveraging reinforcement learning to refine the model’s reasoning capabilities without excessive dependence on labeled datasets.
How DeepSeek-R1 Works
DeepSeek introduces two models:
- R1-Zero: A model trained purely via RL from scratch, without prior SFT. This approach allows the model to develop emergent reasoning behaviors independently.
- R1: A more refined version that undergoes multi-stage training. Initially, it is exposed to a cold-start dataset before reinforcement learning is applied to enhance reasoning efficiency and accuracy.
This innovative training strategy fosters emergent reasoning capabilities, allowing DeepSeek-R1 to outperform many existing models in STEM-related tasks. Notably, the model maintains concise and precise outputs, avoiding the common tendency of LLMs to produce excessive or redundant information.
Key Findings and Performance Benchmarks
The release of DeepSeek-R1 has been accompanied by impressive performance metrics across multiple benchmarks. The model competes favorably with OpenAI’s latest releases, demonstrating superior or comparable results in:
- MMLU (Massive Multitask Language Understanding)
- MMLU-Pro, a more advanced variant focusing on complex reasoning
- GPQA Diamond, which evaluates factual and analytical capabilities
Additionally, DeepSeek-R1 excels in document analysis, fact-based queries, and mathematical problem-solving, showcasing its ability to navigate challenging reasoning tasks effectively. Its unique RL-based approach enables it to maintain logical consistency while avoiding the pitfalls of verbosity that affect many traditional LLMs.
Why DeepSeek-R1 Is a Game-Changer
DeepSeek-R1's breakthrough has significant implications for the AI landscape:
- Challenges Conventional Training Methods: By demonstrating that reinforcement learning alone can produce highly capable reasoning models, DeepSeek-R1 questions the necessity of extensive supervised datasets. This shift could lead to more efficient model training approaches that require fewer human-labeled samples.
- Enhances STEM and Coding Capabilities: The model’s strong performance in scientific and mathematical domains positions it as a powerful tool for research, education, and professional applications where deep reasoning is required.
- Encourages Open Innovation and Competition: The AI industry is already witnessing heightened competition in response to DeepSeek-R1’s success. Companies like Meta, Google, and OpenAI are reportedly scrambling to analyze and replicate DeepSeek’s methodologies.
- Potential Cost Efficiency: With reinforcement learning proving to be an effective alternative to supervised fine-tuning, the cost of developing high-performance LLMs could decrease. This is particularly relevant in an era where AI model training expenses have skyrocketed.
Industry Reactions and Impact on AI Competitors
The release of DeepSeek-R1 has sent shockwaves through the AI community, prompting discussions about its implications for major industry players:
- Meta’s AI Division in Panic Mode? Internal reports suggest that Meta engineers are urgently analyzing DeepSeek’s innovations, attempting to integrate similar techniques into their own models. Concerns have emerged regarding the efficiency of Meta’s AI research investments, especially given DeepSeek’s relatively smaller budget.
- OpenAI, Google, and Anthropic Under Pressure: DeepSeek’s advancements have intensified the race for superior LLMs. Given its success in reinforcement learning-based reasoning, competitors may be forced to reconsider their reliance on traditional supervised fine-tuning.
- NVIDIA’s Hardware Market May Be Affected: If DeepSeek’s training methods prove significantly more efficient, demand for extensive GPU clusters may decrease. This could impact NVIDIA’s revenue from AI-focused data centers.
- Chinese AI Industry Strengthens: DeepSeek’s success highlights China’s growing influence in AI development. Reports indicate that DeepSeek’s CEO has met with Chinese government officials, potentially securing substantial funding for future advancements. This development raises strategic concerns in the ongoing global AI competition.
Controversies and Challenges
While DeepSeek-R1’s achievements are widely recognized, certain concerns have been raised:
- Intellectual Property and Model Training Ethics: Some industry observers speculate whether DeepSeek’s models may have been influenced by OpenAI’s methodologies. Questions remain about the ethical implications of AI model distillation and training on publicly available outputs.
- Chinese Government Involvement: The AI industry is closely monitoring DeepSeek’s relationship with the Chinese government. Some critics argue that its models may be subject to state censorship, raising concerns about bias in outputs.
- Verification of Performance Claims: As with any major AI breakthrough, independent verification of DeepSeek-R1’s performance will be crucial. While the published benchmarks are impressive, industry leaders will likely seek further validation before fully acknowledging its superiority.
Future Prospects and What’s Next for AI Development
DeepSeek-R1’s release marks a turning point in AI research, with several potential directions for future advancements:
- Further Refinement of RL-Only Models: If reinforcement learning continues to yield strong reasoning capabilities, future LLMs may increasingly adopt this approach, reducing reliance on supervised datasets.
- Expansion into Real-World Applications: DeepSeek-R1’s proficiency in coding, document analysis, and STEM reasoning suggests vast potential for integration into professional tools, educational platforms, and research environments.
- Increased Investment in AI Innovation: Given the intense competition sparked by DeepSeek’s advancements, companies worldwide will likely accelerate AI research funding to stay competitive.
- Potential Acquisition or Strategic Partnerships: With DeepSeek’s rapid rise, speculation is growing about whether major tech firms might attempt to acquire the company or form strategic alliances to leverage its expertise.
Conclusion
DeepSeek-R1 represents a major leap in AI development, proving that reinforcement learning alone can cultivate sophisticated reasoning abilities in LLMs. Its strong performance in STEM fields, efficient training methodology, and competitive benchmarks have set a new standard in the industry. As global AI players scramble to respond, the emergence of DeepSeek-R1 signals a transformative moment in the evolution of large language models. Whether through replication, competition, or strategic adaptation, the impact of DeepSeek-R1 will undoubtedly shape the future of AI research and development for years to come.